Trends and Challenges in Conversational Artificial Intelligence
Conversational artificial intelligence (AI) is already present in many families’ living rooms, cars, and online shopping experiences. Chatbots, voice assistants, smart speakers, interactive voice recognition systems: all of these are examples of conversational AI. It’s an area that’s attracting significant investment given the increased accessibility it offers through enhanced customer experiences.In the simplest of terms, conversational AI can be defined as the interaction between humans and machines. It recognizes speech and text, intent, and various languages in order to mimic natural language or human conversation. Conversational AI solutions can complete repetitive tasks that humans usually do, saving money and time and freeing up humans to work on higher-level strategic endeavors.As one of the fastest-growing fields within the machine learning (ML) space, conversational AI isn’t without its challenges. But with smart workflow planning and strategic infrastructure in place, it can be one of the most profitable areas for businesses to invest in.
Trends to Watch in Conversational AI
Within the conversational AI field, there are several key trends and shifts taking place.
Increasing Adoption of Digital Assistants
Digital assistants are experiencing a steady increase in adoption, with a high growth rate of 34% year over year. These include smart speakers, smart home apps, and other technology-driven voice commands (for example, Amazon Alexa or Google Assistant). Predictions show that in the next two years, one-third of the US population will use voice assistants.
AI Powering In-car Experience
A driver ideally needs to keep her hands on the wheel while navigating her vehicle, making voice the natural solution to perform tasks safely while in motion. Car manufacturers are already enhancing the in-car experience using voice assistance features; in some models, you can ask, “What’s the weather like in Beijing?” and receive an immediate, accurate answer.Car manufacturers are also adding facial recognition features to understand more about the driver and what they need for an ideal driving experience.
Customer Service Integrating AI
In the coming years, AI will be a mainstream investment for companies looking to improve customer experience. According to Gartner, 47% of organizations will leverage chatbots in the next couple of years, while 40% will deploy virtual assistants. This is partly a cost-savings measure, but also a response to increasing customer demands for personalization and immediate resolution to issues. Employing a virtual assistant also helps businesses scale quickly, as these chatbots are cheaper and faster than their human counterparts.
Challenges in Conversational AI
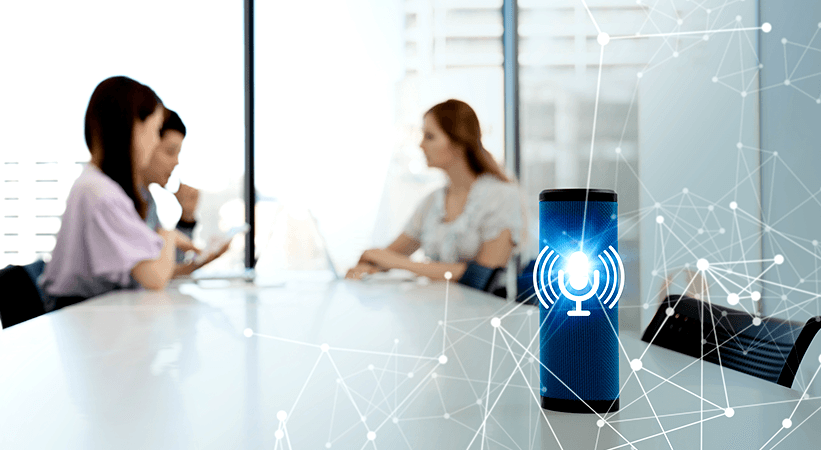
Conversational AI has many of the same challenges that AI development in general poses. Bias and diversity are top of mind and must be tackled head-on. Where these elements play a critical role is in the data used to train the model. Training data for voice assistants, in particular, must have the depth and breadth to cover different dialects, accents, and languages, as the way people across the globe speak is highly varied.When training data is insufficient, the chance of failure increases significantly. For example, a study conducted on popular automatic speech recognition technology recently discovered much higher error rates for African American speakers than Caucasian speakers. These technologies, and the people they serve, would benefit from the far greater representation of African American speakers in their training data. If representation had been addressed in the early phases of these projects, the discrepancies might not be so dramatic, and the customer experience would be less affected.Any time data is involved, privacy and security of that data should also be a primary consideration. Before building AI, companies should create data governance policies to protect sensitive data and ensure they’re sourcing that data ethically.Another point to consider before starting an AI journey is the production scale and ML data pipelines. Building and automating pipelines enables companies to continue to tune and train their model even after deployment, as models will continuously encounter edge cases, new users, and scenarios. Introducing human-in-the-loop is an ideal method for monitoring model performance and providing ground truth accuracy.These challenges aren’t trivial and require ongoing initiative. By asking the right questions around ethics and data upfront, companies in the conversational AI space will have a higher likelihood of satisfying customers across various geographies, cultures, and languages.
Building Workflows for Conversational AI
Constructing a clear project workflow is one of the first steps of the model build process. When designing a conversational AI workflow, remember that the training data preparation stage is the most critical component to get right. This step includes collecting data, labeling it, training your model using that data, and analyzing the outputs. The vast majority of time spent on AI projects is devoted to the training data preparation phase, so companies need to have the right tools and processes in place to achieve success at this critical juncture.Typically, conversational AI will perform the following sequence of events during one interaction with a human:
- Speech-to-text conversion: AI converts the raw audio file of what a customer says into text.
- Natural language understanding (NLU): AI analyzes and processes text to create actionable instructions.
- Content Relevance: AI returns optimal info that can help the customer.
A sample workflow scenario of building a conversational AI model might include, for example, an in-car virtual assistant. A training data preparation workflow may look something like this:Step 1. Collect audio data with customer commands and include quality assurance steps to ensure the data is high-quality and accurate. Rework any data that’s low-quality.Step 2. Segment audio clips to detect which part of the clip is speech, background noise, or music.Step 3. Transcribe audio clips to convert them into text.Step 4. Annotate and label the text to identify intent and achieve an understanding of natural language. Assign class labels for different tokens to each of the words in the sentence.Step 5. Train your model on these data types so it can understand the subject of a voice command, as well as the context and intent behind it.Most companies will employ crowd workers from various geographies and languages to handle the massive amounts of annotation work required for this workflow and maximize diversity in their models.
How a Data Partner Can Help
The previous example was a simple workflow or data pipeline. These steps can become increasingly complicated as your model grows in complexity. In any case, you’ll want to look for a data platform and partner that supports a wide variety of use cases and can help you automate your pipeline for use during the model build, deployment, and beyond. This will enable you to scale quickly and handle model drift. Success in conversational AI, and AI as a whole, is achievable for companies that keep quality and scalability in mind and those that build the right processes, infrastructure, and toolsets. Learn more about how Appen can help.