Appen joined the International Conference on Learning Representations (ICLR) as an exhibitor. This year’s conference spotlighted cutting-edge advancements including large language models (LLMs), multimodal AI, agentic systems, and embodied AI. As these systems scale in complexity and capability, the research community is not only pushing the frontiers of architecture and pretraining, but also rigorously exploring challenges in alignment, evaluation, and real-world deployment.
Our conversations at the booth and social sessions offered direct insight into the evolving priorities of AI labs, startups, and enterprise teams, and where high-quality human-in-the-loop data pipelines play a critical role.
AI Safety in Practice: Bridging Theory and Real-World Challenges
In this social, our team shared findings from our recent work in adversarial prompting and explored practical strategies to build safer, more accountable models.
- Generative AI models still frequently display undesired behaviours
In our adversarial prompts evaluation dataset, we assessed the performance of top open- and closed-source models including GPT-4o, Claude 3.7 Sonnet, Llama 3.3 70B Instruct, and DeepSeek R. The results show results of adversarial prompting techniques like virtualisation, sidestepping, and prompt injection, and highlight that substantial safety performance gaps—even in models with state-of-the-art scale and compute. - Human-in-the-loop is critical in ensuring model safety
While synthetic data and automated benchmarks are widely used in AI training and evaluation, most attendees noted failure cases in edge scenarios and subjective evaluations. There is increasing consensus that human annotation is still essential for nuanced tasks like reasoning, safety, and alignment. - More real-world benchmarks for AI safety are necessary
Current evaluation frameworks often fail to capture the complexity of real-world deployment scenarios where models have diverse end users and use cases. Developing dynamic LLM evaluation benchmarks that evolve alongside models and better represent the challenges of production environments requires more customized approaches targeted to enterprise scenarios. - Responsible AI and governance are key considerations for enterprises, government, and technology builders
Organizations are increasingly seeking practical AI safety frameworks that balance innovation with appropriate guardrails for AI deployment. Collaboration between technical teams, policy experts, and domain specialists is essential for developing governance structures that are both effective and adaptable to rapidly evolving AI capabilities.
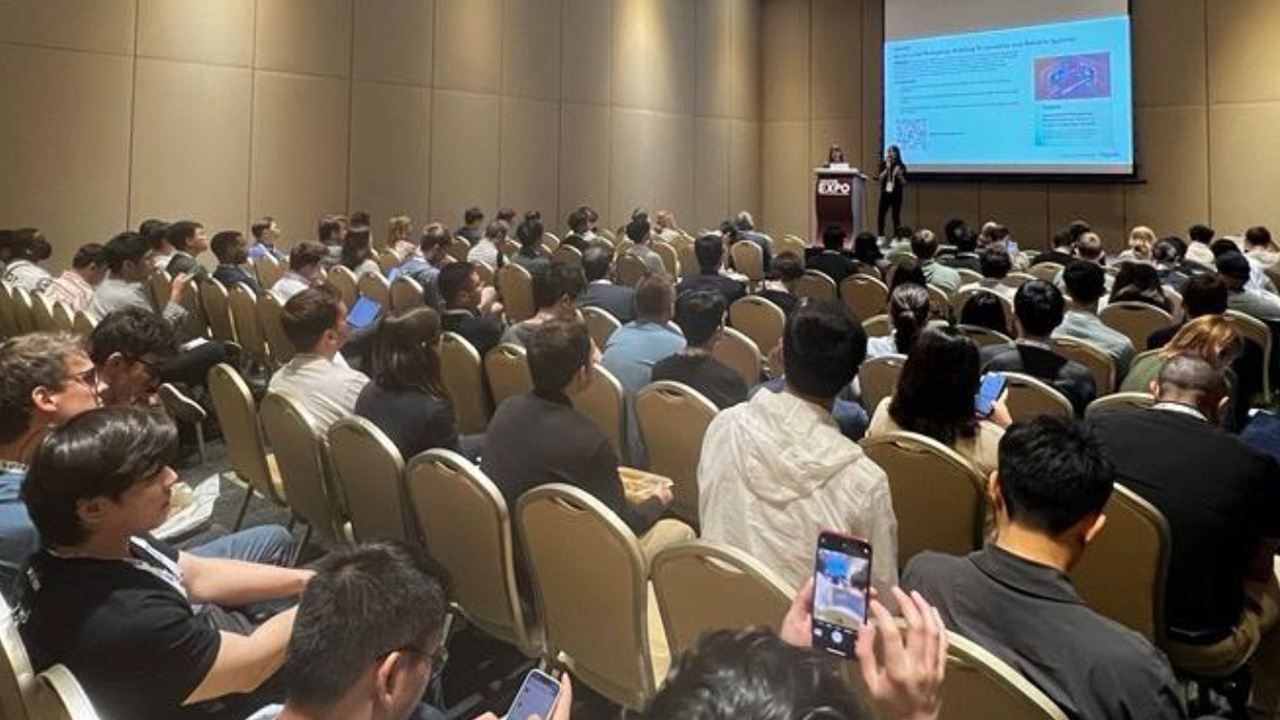
Global AI Systems: Inclusive and Culturally Aware AI
In this social, we explored how cultural and linguistic diversity must be embedded into AI development for models to succeed across geographies and user contexts.
- Language ≠ Culture and ignoring that creates real risk
Many AI systems are multilingual, but not all are culturally fluent. We discussed examples where sentiment detection, design generation, or accessibility tools failed because they lacked regional and contextual nuance. From localized misinformation to stylized manga, multilingual AI models must reflect more than just translated words, they need grounded cultural understanding. - Cultural context enhances model trust, usability, and performance
Through case studies like Japanese manga image description and graphic design evaluations across 25 languages, we demonstrated how native-speaking experts improved the accuracy and cultural relevance of model outputs. These projects required both linguistic proficiency and lived cultural familiarity, showing that synthetic or templated data pipelines fall short in these domains. - Real-world use cases require localized knowledge at scale
Our misinformation detection program, running across 20 countries for over 6 years, showed how dynamic, fast-changing data needs regionally trained contributors. With 30M+ jobs completed in the past year, it’s clear that scaling global systems demands ongoing human-in-the-loop input, tailored workflows, and cultural calibration. - Enterprises are seeking inclusive AI frameworks
Global enterprises increasingly recognize that model fairness, safety, and usability must include representation from diverse geographies and communities. Beyond compliance, inclusion has become a performance factor, shaping AI outputs that feel relevant and trustworthy for users around the world.
Looking Ahead
Appen’s heritage - spanning 28+ years, over 1M contributors, and 500+ languages – stood out to many researchers and developers we spoke to. From rapid-turnaround multilingual red-teaming to multimodal data collection for embodied agents, our work in these areas is only accelerating.
As the research community continues to push boundaries, we’re proud to support AI systems that are not only intelligent but safe, inclusive, and grounded in the complexity of the real world.
Want to learn more or partner with us?
Let’s talk about your next model evaluation or alignment project. You can find more about our capabilities here.