Adobe Stock: Improves Search Relevance of Massive Asset Profile
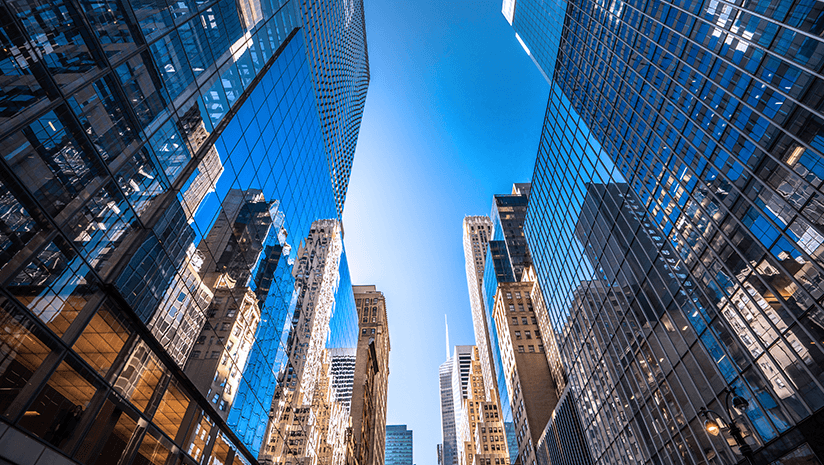
The Company
Adobe is the global leader in creative software. You’d be hard-pressed to find a professional designer who isn’t well-versed in Photoshop, Illustrator, or InDesign, but Adobe has dozens of other offerings, spanning not just the design space, but e-signatures, analytics, marketing, stock photography, and more. They are a true pioneer in the creative software space, constantly innovating on their product suite while streamlining the entire design process.
The Challenge
One of Adobe’s flagship offerings is Adobe Stock, a curated collection of high-quality stock imagery. The library itself is staggeringly large: there are over 200 million assets (including more than 15 million videos, 35 million vectors, 12 million editorial assets, and 140 million photos, illustrations, templates, and 3D assets). Every one of those assets needs to be discoverable.
Adobe has plenty of metadata for their searches that are provided by content uploaders. They provide their own information like the objects in the image, the mood, the aesthetic, and more. But that isn’t quite enough. For starters, those user-provided tags can sometimes be over-broad or incorrect. And most importantly, they don’t speak to the way end-users actually utilize these images in marketing collateral.
For example, many Adobe Stock customers are looking for images that allow them to place text over an image. That requires a certain type of image, where copy can sit on a clean background free of busy extra objects. These types of images are quite popular for marketers looking to create clean, vibrant collateral.
The issue is that while these particular images tend to be some of the most frequently downloaded assets, that feature isn’t something that exists in the metadata Adobe’s uploaders provide. To better serve their customers, Adobe needed to create a model that could find key attributes in images like copy space or object isolation.
The Solution
Adobe needed highly accurate training data to create a model that could surface these subtle attributes in both their library of over a hundred million images, as well as the hundreds of thousands of new images that are uploaded every day. They used our platform to facilitate the drawing of polygons over areas that could best be used for copy blocks (think large white spaces or tabletops). For example:
Those accurately annotated polygons helped inform their models about what those spaces actually look like. They’ve run similar workflows for categorizing object isolation as well, another popular image type that’s hard to truly classify with metadata.
The Result
That training data powers models that help Adobe serve their most valuable images to their massive customer base. Instead of scrolling through pages of similar images, users can find the most useful ones quickly, freeing them up to start creating powerful marketing materials.
Adobe Stock is a perfect example of how to leverage a massive catalog of unique data and create models that make customers happy. These human labels don’t supersede the metadata or color attributes that Adobe can detect without annotators. Rather, combining human-in-the-loop machine learning practices makes their models more effective, more powerful, and more useful.